Identifying birds, butterflies, and wildflowers with a snap
September 13, 2014 | categories: Biology, Python, Deep Learning, Programming, Machine Learning, Computer Vision | View Comments
It's been a while since I started my little adventure of building an image recognition pipeline that would reliably identify animal and plant species from photos. I'm pretty happy with what the results are so far, and these days I'm even successfully scratching my own itch with it. Which is how I came up with the idea in the first place: I suck at identifying birds, butterflies, and plants, but I want to learn about them. And knowing an animal or plant's name is really the gateway to learning more about it.
So here's a painless way of identifying these species. Say you want to identify one bird that you see (and you're lucky to have a camera with a big zoom with you). You take a photo of it and then send it to @id_birds on Twitter using Twitter's own image upload. After a minute or two, you'll get the automatic reply, with hopefully the right species name. @id_birds can with a remarkably high accuracy identify yours between the 256 different birds that it knows.
There's two other bots that are also available to use for free: @id_butterflies knows around 50 butterfly species, and @id_wildflowers around 200 wildflowers. All three bots know species occurring natively in Europe only, at least for now.
"But does it actually work?" I hear you say. And the answer is: it works pretty well, especially if you send good quality photos where the animal or plant is clearly visible.
Seeing is believing though, so to prove that it works well, let's try and identify the ten birds that are in The Wildlife Trust's river bird spotter sheet.
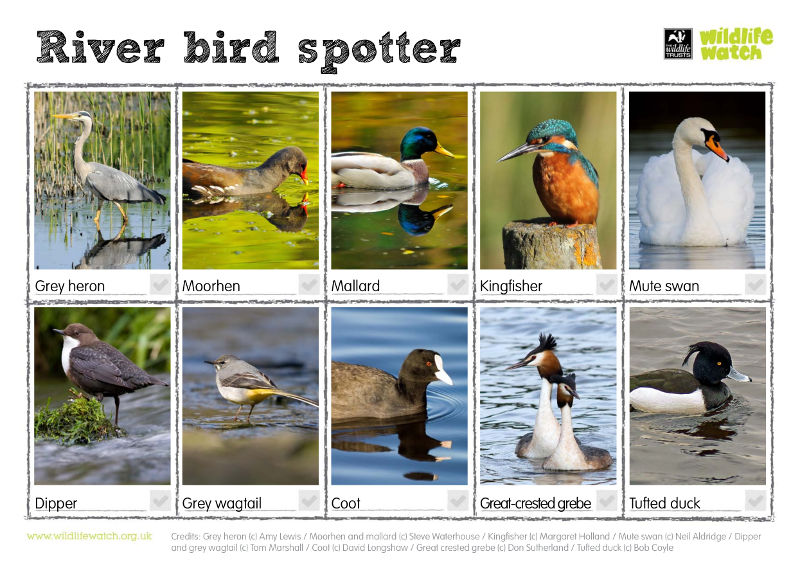
Image credits: Grey heron (c) Amy Lewis / Moorhen and mallard (c) Steve Waterhouse / Kingfisher (c) Margaret Holland / Mute swan (c) Neil Aldridge / Dipper and grey wagtail (c) Tom Marshall / Coot (c) David Longshaw / Great crested grebe (c) Don Sutherland / Tufted duck (c) Bob Coyle
Let's start with the first bird, a grey heron. To the left we have me addressing the Twitter bot and sending the image. To the right is @id_birds' reply. And it's correct! Ardea cinerea is indeed the scientific name for the grey heron:
(If you're not seeing the tweets below, I suggest you view this on my blog.)
@id_birds hey can you ID me this bird from @wildlifetrust's river birds spotter sheet? pic.twitter.com/z5JnE3WIb3
— Daniel Nouri (@dnouri) September 13, 2014
@dnouri
1. Ardea cinerea http://t.co/gGuu8ombMs
2. Anthropoides virgo http://t.co/exqiChamSg
— id_birds (@id_birds) September 13, 2014
Notice how @id_birds will always respond with the two most likely guesses. The second guess here was the demoiselle crane (Anthropoides virgo), which looks sort of similar.
The next bird on the river bird spotter sheet is the common moorhen (Gallinula chloropus). Let's try to ID it:
@id_birds how about this one? pic.twitter.com/9csoNaAaJw
— Daniel Nouri (@dnouri) September 13, 2014
@dnouri
1. Anas platyrhynchos http://t.co/9xoDyXJGMJ
2. Gallinula chloropus http://t.co/ISQ0N7m2Lu
— id_birds (@id_birds) September 13, 2014
And already we have @id_birds' first mis-classification. It thinks that this moorhen is a mallard (Anas platyrhynchos). Only the second guess is correct. Thus so far we're counting one correct, one error.
The next bird we want to identify is incidentally a mallard:
@id_birds pic.twitter.com/x9h89dKkkb
— id_mushrooms (@id_mushrooms) September 13, 2014
@id_mushrooms
1. Anas platyrhynchos http://t.co/9xoDyXJGMJ
2. Coracias garrulus http://t.co/MKJTOTfeOv
— id_birds (@id_birds) September 13, 2014
And the response is correct. Notice how the second guess (Coracias garrulus) seems a little weird. I guess it's the similarities in color here. We don't count the second guesses here though, so it's two correct, one error.
What about the kingfisher? That's a bird with a pretty unique appearance among birds native to Europe. So it's probably one of the easier birds to identify:
@id_birds pic.twitter.com/NNy1t6izLQ
— id_butterflies (@id_butterflies) September 13, 2014
@id_butterflies
1. Alcedo atthis http://t.co/Q1fUKAlYSt
2. Hirundo rustica http://t.co/mtVqrMC8oG
— id_birds (@id_birds) September 13, 2014
And sure enough, @id_birds knows what it is. The latin name for the kingfisher is Alcedo atthis.
The fifth bird on the spotter sheet is the mute swan (Cygnus olor). Everyone probably knows this one, but does @id_birds?
@id_birds and this? pic.twitter.com/RiFk3pP38B
— id_butterflies (@id_butterflies) September 13, 2014
@id_butterflies
1. Cygnus olor http://t.co/jz7DLdqVW2
2. Cygnus cygnus http://t.co/wx8MSFv3sy
— id_birds (@id_birds) September 13, 2014
Yes, the answer is correct. Note that the second guess is also a swan, namely the whooper swan, quite similar in appearance. A good job!
So out of the first five birds we got four correct, not bad. But let's see about the rest of the birds from the spotter sheet.
I'll post the responses to the remaining five birds without further comment. But do note that of the remaining ones, only the coot (Fulica atra) is mis-classified, that's the third one below:
@id_birds I found this one in @wildlifetrust's river birds spotter sheet. Do you know what it is? pic.twitter.com/R9Qf5s2sSf
— id_wildflowers (@id_wildflowers) September 13, 2014
@id_wildflowers
1. Cinclus cinclus http://t.co/u2W2R8NYZX
2. Corvus corone http://t.co/1JjU7HtcSj
— id_birds (@id_birds) September 13, 2014
@id_birds Here's another cute bird from that same spotter sheet. Can you ID it? pic.twitter.com/onxOeALtaL
— Daniel Nouri (@dnouri) September 13, 2014
@dnouri
1. Motacilla cinerea http://t.co/RhZyl5ltwy
2. Motacilla flava http://t.co/TWG5YgTxBQ
— id_birds (@id_birds) September 13, 2014
@id_birds pic.twitter.com/qYVqQGKa4I
— id_wildflowers (@id_wildflowers) September 13, 2014
@id_wildflowers
1. Gallinula chloropus http://t.co/ISQ0N7m2Lu
2. Podiceps nigricollis http://t.co/WgflyFJyCU
— id_birds (@id_birds) September 13, 2014
@id_birds Can you ID this as Podiceps cristatus? pic.twitter.com/jQwS5cjuEi
— id_wildflowers (@id_wildflowers) September 13, 2014
@id_wildflowers
1. Podiceps cristatus http://t.co/JzrmePWuf9
2. Branta ruficollis http://t.co/X6fCvqF0xo
— id_birds (@id_birds) September 13, 2014
@id_birds here's the last one: a tufted duck (Aythya fuligula) pic.twitter.com/S8VwHd6rry
— id_butterflies (@id_butterflies) September 13, 2014
@id_butterflies
1. Aythya fuligula http://t.co/n4O4tTIwtv
2. Aythya marila http://t.co/H9g0mXy6Eu
— id_birds (@id_birds) September 13, 2014
So all in all we have two mis-classifications out of ten. That's 8 out of 10 accuracy, which is pretty remarkable when you consider that the differences between these 256 different birds species can sometimes be minuscule. As an example, compare the grey wagtail (Motacilla cinerea) from above with the yellow wagtail. According to Wikipedia, the grey wagtail "looks similar to the yellow wagtail but has the yellow on its underside restricted to the throat and vent."
Of course ten picture is a tiny test set, but the score roughly matches what I see with the much larger test set that I use for development, so it's cool. :-)
I'll write about how the computer vision works under the hood another time (but here's a hint: it's based deep learning). We call the stack that we've developed and that's running behind @id_birds and the other bots PhotoID. So far PhotoID has proven to have very competitive performance and be remarkably flexible to use for different image classification problems.
Let's conclude this post with two other IDs, one from @id_butterflies, the other from @id_wildflowers:
#WansteadFlats It's an upside down world sometimes. Maybe a Meadow Brown? @id_butterflies @wildlife_uk pic.twitter.com/yDnkTUTMO8
— TheNatureOfTheFlats (@RoseStephensArt) August 22, 2014
@id_butterflies
1. Maniola jurtina http://t.co/4YGYG6WQHc
2. Cupido minimus http://t.co/2hbLKCYzpF
— id_butterflies (@id_butterflies) August 22, 2014
Can @id_wildflowers identify this wildflower photographed by @LornaCrowcroft?
Wondering what it is? See the reply. pic.twitter.com/WI31e7aN8x
— id_wildflowers (@id_wildflowers) September 4, 2014
@id_wildflowers
1. Solanum nigrum http://t.co/kvMbrC7KB4
2. Cyclamen hederifolium http://t.co/mcZHVmRecq
— id_wildflowers (@id_wildflowers) September 4, 2014
Happy ID'ing!
(Plug: Feel free to contact me through my company if you have a challenging image recognition problem that you want us to help solve.)
Using deep learning to listen for whales
January 10, 2014 | categories: Python, Biology, Programming, Bioacoustics, Machine Learning | View Comments
Since recent breakthroughs in the field of speech recognition and computer vision, neural networks have gotten a lot of attention again. Particularly impressive were Krizhevsky et al.'s seminal results at the ILSVRC 2012 workshop, which showed that neural nets are able to outperform conventional image recognition systems by a large margin; results that shook up the entire field. [1]
Krizhevsky's winning model is a convolutional neural network (convnet), which is a type of neural net that exploits spatial correlations in 2-d input. Convnets can have hundreds of thousands of neurons (activation units) and millions of connections between them, many more than could be learned effectively previously. This is possible because convnets share weights between connections, and thus vastly reduce the number of parameters that need to be learned; they essentially learn a number of layers of convolution matrices that they apply to their input in order to find high-level, discriminative features.
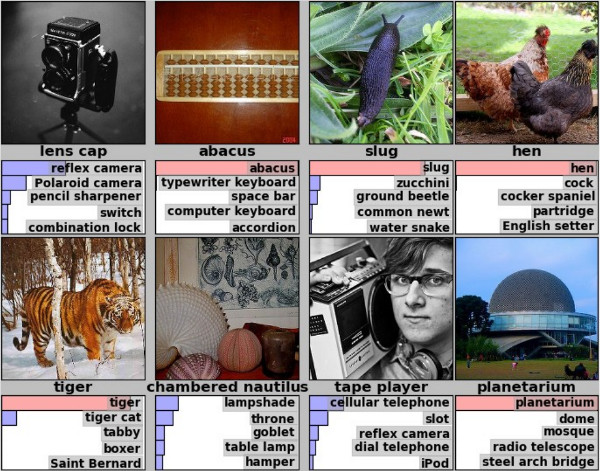
Figure 1: Example predictions of ILSVRC 2012 winner; eight images with their true label and the net's top five predictions below. (source)
Many papers have since followed up on Krizhevsky's work and some were able to improve upon the original results. But while most attention went into the problem of using convnets to do image recognition, in this article I will describe how I was able to successfully apply convnets to a rather different domain, namely that of underwater bioacoustics, where sounds of different animal species are detected and classified.
My work on this topic began with last year's Kaggle Whale Detection Challenge, which asked competitors to classify two-second audio recordings, some of which had a certain call of a specific whale on them, and others didn't. The whale in question was the North Atlantic Right Whale (NARW), which is a whale species that's sadly nearly extinct, with less than 400 individuals estimated to still exist. Believing that this could be a very interesting and meaningful way to test my freshly acquired knowledge around convolutional neural networks, I entered the challenge early, and was able to reach a pretty remarkable Area Under Curve (AUC) score of roughly 97% after only two days into the competition. [2]
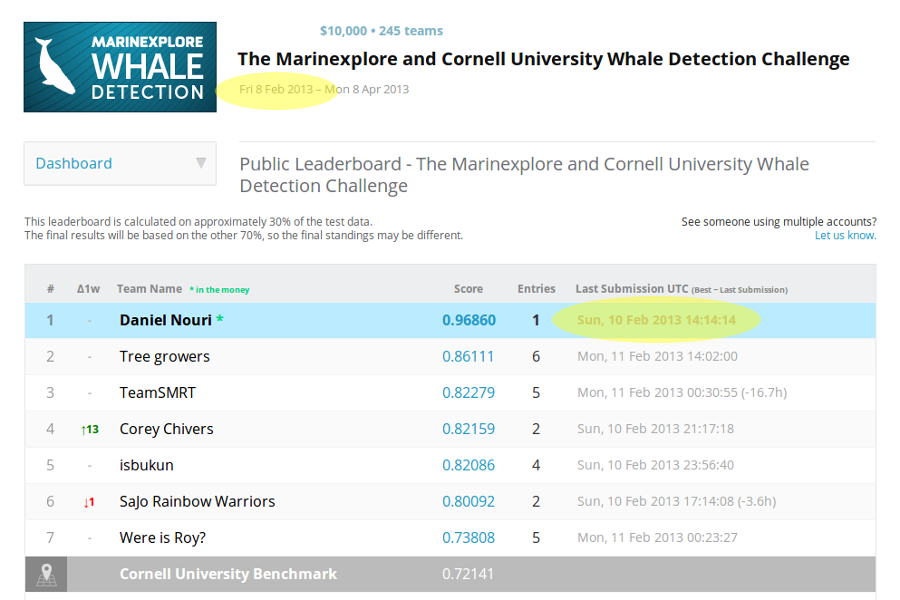
Figure 2: The Kaggle leaderboard after two days into the competition.
The trick to my early success was that I framed the problem of finding the whale sound patterns as an image reconition problem, by turning the two-second sound clips each into spectrograms. Spectrograms are essentially 2-d arrays with amplitude as a function of time and frequency. This allowed me to use standard convnet architectures quite similar to those Krizhevsky had used when working with the CIFAR-10 image dataset. With one of few differences in architecture stemming from the fact that CIFAR-10 uses RGB images as input, while my spectrograms have one real number value per pixel, not unlike gray-scale images.
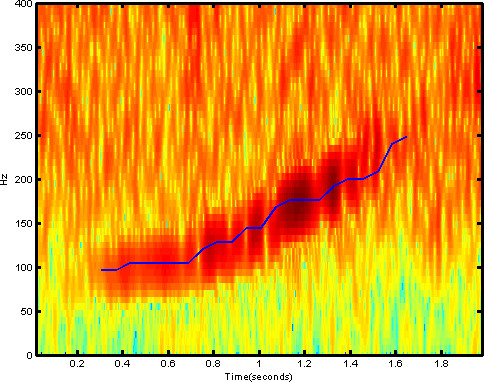
Figure 3: Spectrogram containing a right whale up-call.
Spurred by my success, I registered for the International Workshop on Detection, Classification, Localization, and Density Estimation (DCLDE) of Marine Mammals using Passive Acoustics, in St Andrews. A world-wide community of scientists meets every two years at this workshop to discuss the latest developments in using passive acoustics (listening for sounds) to detect and track marine mammals.
That there was such a breadth of research around this topic was entirely new to me, and it was fascinating to learn about it. Another thing that I've since learned is that this breadth is sadly dwarfed by the amounts of massive underwater noise that humans produce today, through shipping, oil exploration, and military sonar. And this noise severely affects the lives of animals for which "listening is as important as seeing is for humans – they communicate, locate food, and navigate using sound."
The talk that I gave at the DCLDE 2013 workshop was well received. In it, I elaborated how my method relied on little to no problem-specific human engineering, and therefore could be easily adapted to detect and classify all sorts of marine mammal sounds, not just right whale up-calls.
At DCLDE, the execution speed of detection algorithms was frequently quoted as being x times faster than real-time, with x often being a fairly low number around 1 to 10. My GPU-powered implementation turned out to be on the faster side here: on my workstation, it detects and classifies sounds 700x faster than real-time, which means it runs detections on one year of audio recordings in roughly twelve hours, using only a single NVIDIA GTX 580 graphics card.
In terms of accuracy, it was somewhat hard to get an idea of which of the algorithms presented really worked better than others. This had two reasons: the inconsistent use of reliable metrics such as AUC and use of cross-validation, and a lack of standard datasets that everyone could test their algorithms against. [3]
However, it should be mentioned that good datasets are a bit tricky to come by in this field. The nature of hydrophone recordings is that the signal you're listening for could be generated a few meters away, or many kilometers, and therefore be very faint. Plus, recordings often contain a lot of ambient noise coming from cargo ships, offshore drilling, hydrophone cable flutter, and the like. With the effect that often it's hard even for a human expert to tell if the particular sound they're listening to is a vocalization of the mammal they're looking for, or just noise. Thus, analysts will often label segments as unsure, and two analysts will sometimes even give conflicting labels to the same sound.
Four NARW up-calls that are easy to detect.
(Here's a much messier example.
And some more fascinating recordings of marine mammals.)
This leads to a situation where people tend to ignore noisy sounds altogether, since if you consider them, predictions become difficult to verify manually, and good training examples harder to collect. But more importantly, when you ignore sounds with a bad signal-to-noise ratio (SNR), your algorithms will have an easier time learning the right patterns, too, and they will make fewer mistakes. As it turns out, noise is often more of a problem for algorithms than it is for human specialists.
The approach of ignoring sounds with a bad SNR seems fine until you're in a situation where you've put a lot of effort into collecting recordings, and then they turn out to be unusually noisy, and trying to adjust your model's detection threshold yields either way too many false positives detections, or too many calls are missed.
One of the very nice people I met at DCLDE was Holger Klinck from Oregon State University. He wanted to try out my convnet with one of his lab's "very messy" recordings. Some material that his group at OSU had collected at five sites near Iceland and Greenland in 2007 and 2008 had unusually high levels of noise in them, and their detection algorithms had maybe worked less than optimal there.
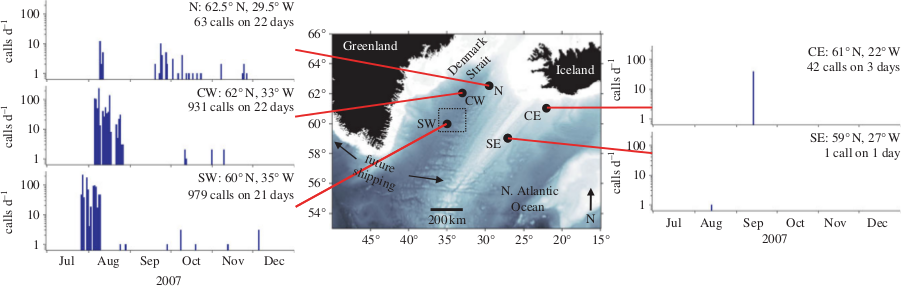
Figure 4: "Locations of passive acoustic moorings near Iceland and southern Greenland (black spots), and the number of right whale upcalls detected per day in late 2007 at the five sites." Taken from [4]. Note the very low number of calls detected at the CE and SE sites.
I was rather amazed when a few weeks later I had a hard disk from OSU in my hands containing in total many years of hydrophone recordings from two sites near Iceland and four locations on the Scotian Shelf. I dusted off the model that I had used for the Kaggle Whale Detection Challenge and quite confidently started running detections on the recordings. Which is when I was in for a surprise: the predictions my shiny 97% model made were all really lousy! Very many obvious non-whale noises were detected wrongly. How was it possible?
To solve this puzzle, I had to understand that the Kaggle Whale Detection Challenge's train and test datasets had a strong selection bias in them. The tens of thousands of examples that I had used to train my model for the challenge were unrepresentative of all whale, and particularly, a lot of similar-sounding non-whale sounds out there. That's because the Kaggle challenge's examples were collected by use of a two-stage pipeline, where an automated detector would first pick out likely candidates from the recording, only after which a human analyst would label them with true or false. I realized that what we were building in the Kaggle challenge was a classifier that worked well only if it had a certain detector running in front of it that would take care of the initial pass of detection. My neural net had thus never seen during training anything like the sounds that it mistook for whale calls now.
If I wanted my convnet to be usable by itself, on continuous audio recordings, and independently of this other detector, I would have to train it with a more balanced training set. And so I ditched most of the training examples I had, and started out with only a few hundred, and trained a new model with them. As was expected, training with only few examples left me with a pretty weak model that would overfit and make lots of obvious mistakes. But this allowed me to pick up the worst mistakes, label them correctly, and feed them back into the system as training examples. And then repeat that. (A process that Olivier Grisel later told me amounts to active learning.)
Many (quite enjoyable) hours of listening to underwater sounds later, I had collected some 2000 training examples this way, some of which were already pretty tricky to verify. And luckily, the newly trained model started to make pretty good predictions. When I sent my results back to Holger, he said that, yes, the patterns I'd found were very similar to those that his group had found for the Scotian Shelf sites!
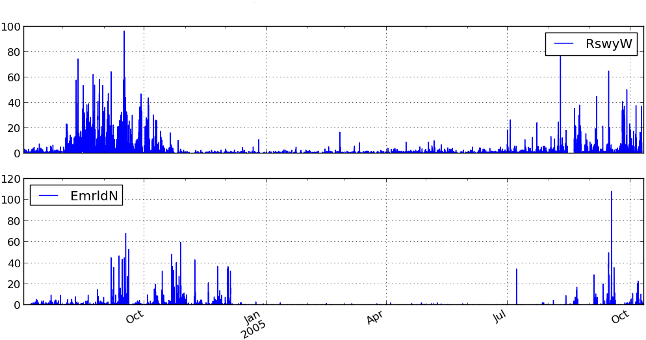
Figure 5: Number of right whale up-call detections per hour at two sites on the Scotian Shelf, detected by the convnet. The numbers and seasonal pattern match with what Mellinger et al. reported in [5].
The OSU team had used a three-stage detection process to produce their numbers. Humans verified in phases two (broadly) and three (in more detail) the detections that the algorithm came up with in phase one. Whereas my detection results came straight out of the algorithm.
A case-by-case comparison still needs to happen, but the similarities of the overall call patterns suggests that the convnet reaches comparable performance, but without the need for human analysts to be part of the detection pipeline, making it potentially much more time-efficient to use in practice.
What's even more exciting is that the neural net was able to find right whale up-calls at the problematic SE site near Iceland, where previously no up-calls could be detected due to high noise levels.
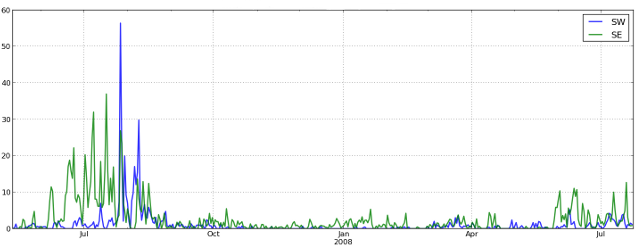
Figure 6: NRW up-call detections per day at sites SW and SE near Iceland, detected by the convnet. The patterns at the SW site match roughly with what was reported in [4], while no calls could be identified previously at the SE site (cf. Figure 4).
Another thing we're currently looking into is wheter or not the relatively small but constant number of calls that the convnet detected during Winter season are real, or if they're false positives. Right whales are not known to hang around so high up North during that time of the year, so proving that would constitute significant news for people studying the migration routes of these whales.
(Comments also on Hacker News.)
[1] | For a more detailed history and recent developments around neural nets, see this article in Nature: "Computer science: The learning machines". |
[2] | See the mention of my results in this Wired article: "Wanted: Right Whale Caller ID". |
[3] | For a comparison of machine learning algorithms in use, see: Mellinger DK, et al. 2007. An overview of fixed passive acoustic observation methods for cetaceans. Oceanography 20:36–45. |
[4] | (1, 2) Mellinger DK, et al. 2011. Confirmation of right whales near a historic whaling ground east of Southern Greenland. Biol Lett 7:411−413 |
[5] | Mellinger DK, et al. 2007. Seasonal occurrence of North Atlantic right whale (Eubalaena glacialis) vocalizations at two sites on the Scotian Shelf. Mar. Mamm. Sci. 23, 856–867. |